Roadmap: Here’s how to navigate risks and ethical concerns in advanced AI technologies
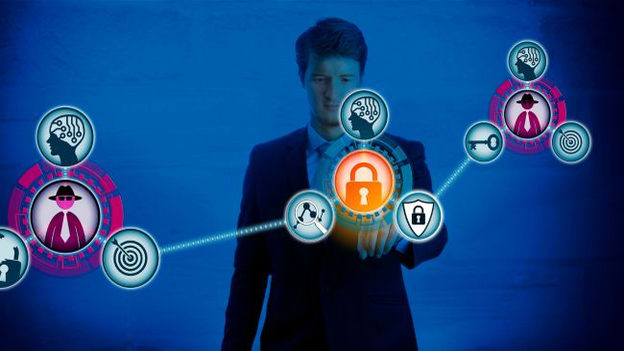
Artificial Intelligence (AI) has emerged as a revolutionary force with the potential to transform multiple industries and bring about significant advancements. It promises enhanced efficiency, accuracy, and innovation in fields ranging from healthcare to finance.
However, as a powerful technology, AI also raises risks and ethical concerns that necessitate careful attention and addressing.
In an interaction with People Matters, Dattaraj Rao, Chief Data Scientist at Persistent Systems, delves into the potential dangers associated with advanced AI technologies and the measures that can be taken to mitigate negative consequences.
Excerpts
The potential risks of AI
Like any other technology, AI can also be used to cause harm or lead to unintentional risks. These risks could be bias in models making critical decisions like loan approval or disease diagnosis. For example, when critical decisions like credit approval get biased by factors like gender, it may cause resentment towards this technology. This has given rise to the field of model risk management, where studies are conducted on risks caused by incorrect decisions made by ML models.
Mitigating negative consequences
We highly recommend organisations embrace responsible AI principles around reproducibility, transparency, accountability, security, and privacy. AI/ML models built should be reproducible, and others should recreate the same results on the same version of data. Having a data catalog is highly recommended to ensure good quality models. These models should have some lineage to source to provide accountability – especially models that search information in databases. Finally, security and privacy are most important, and ML deployment should ensure this with thorough testing and attention to data leakage.
Ethical considerations in AI development
Data used to build AI models should be checked for bias against protected attributes like gender, race, age, etc. If bias is found, corrective measures need to be taken so that it does not percolate into the model while training. After the model is deployed, it is advisable to continuously monitor for data and concept drift, to ensure that the model’s performance is adequate and reflects reality.
Addressing bias and discrimination
The recommended way is to first test the data for bias and make sure it’s free from bias, and if there is bias, apply debiasing techniques. After deploying the model using drift analysis, monitoring the performance is critical to ensure that the model doesn’t fall beyond a specified threshold.
Balancing AI benefits and risks
The key is to adopt responsible AI and clearly define the metrics around fairness, bias, and error analysis. While AI has tremendous benefits, we can make sure the models are free of bias and prejudice using some additional measures. With the help of explainable AI tools, we can validate the response from the model and ensure that the right factors are considered in decision-making. We recommend an explainability report to be continuously analysed by the AI ethics committee to make sure the model is performing as expected.