5 uses of AI that will challenge companies’ ethics
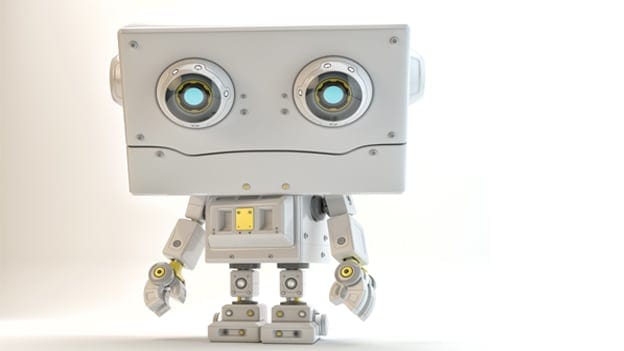
The use of AI in business has boomed in the last few years, and is expected to keep going up as companies make the transition to Industry 4.0. However, alongside ever more powerful implementations of the technology have come increasingly serious ethical concerns. One study by the Capgemini Research Institute found that over 90 percent of organizations believe their use of AI has led to ethical issues in the last two or three years: in healthcare, for example, patients’ personal data has been collected without their consent, and companies in the financial industry have failed to disclose that their decisions are automated.
On top of the issues of privacy and transparency, some applications of AI may inherently be more dangerous than others. In a seminar earlier this month, Kathy Baxter, the head of ethical AI practice at Salesforce, shared five applications of AI that she considers particularly high risk. These applications, in her view, have, or will have, high potential to violate human rights; to enable invasive surveillance; to violate regulations; and to create and spread disinformation.
Facial recognition
Rights groups consider facial recognition to be an extremely invasive surveillance technology. Earlier this month, US-based digital rights advocacy group Fight for the Future launched a campaign to get facial recognition banned from school campuses, saying that the technology violates students’ privacy.
On top of this concern, facial recognition has been found to have high levels of bias and inaccuracy. According to a study carried out by the US National Institute of Standards and Technology, facial recognition algorithms around the world misidentify some ethnic groups up to 100 times more frequently than the average.
“That is not acceptable!” said Baxter. “What technology are we releasing into public spaces that is 100 times more inaccurate for some groups?”
Emotion recognition and prediction
Emotion recognition algorithms are widely used: in security, hiring, education, game development, mental health, and even to help individuals on the autism spectrum improve their social engagement abilities. But various studies have found that the algorithms are not in fact accurate. They rely on recognizing facial expressions, but the link between facial expressions and actual emotion is tenuous at best, according to a scientific review carried out last year. One of the review’s authors, psychology professor Lisa Feldman Barrett, said that while the algorithms might be able to detect a scowl, for example, that does not mean they are detecting anger, simply because a scowl is only an expression of anger about 30 percent of the time.
In other words, emotion recognition algorithms are highly likely to misattribute emotions to people, and in so doing, determine outcomes erroneously—security, legal, career, medical.
“Would you really want outcomes being determined on this basis?” said Barrett. “Would you want that in a court of law, or a hiring situation, or a medical diagnosis, or at the airport ... where an algorithm is accurate only 30 percent of the time?”
Hiring practices
AI is now so widely used for resume and application screening that it has spawned an entire sub-industry of “cheat sheets” and advisory services teaching candidates how to get past automated selection. Some employers even use video-based AI to assess the verbal responses and facial expressions of candidates in pre-recorded videos. But this use, said Baxter, amplifies the same problems that already surfaced in the stand-alone applications of facial and emotional recognition.
Citing the case of Amazon, which notoriously found a gender bias in its AI recruiting tool so severe and pervasive that the company could not eliminate it even after three years of work and finally had to scrap the tool, Baxter pointed out that unchecked bias in an AI model can essentially cause a hiring company to destroy its own candidate pool.
“An AI model [that’s improperly set up] can come back and tell you that the best predictor for a candidate’s success is if the candidate’s name is Jared. Obviously that’s ridiculous!” she said.
Healthcare
Like facial recognition, healthcare AI is highly prone to inaccuracy for some populations, especially minorities or less studied groups. In this field, however, Baxter pointed out that the bias in AI frequently mirrors the bias in professional knowledge. For example, doctors in the US are not trained to recognize skin cancer in darker-skinned people, and this is reflected in the way medical AI is trained.
“What that means is, certain populations are under-served,” she said, and quoting AI activist Joy Buolamwini, who has done extensive work on identifying and mitigating bias in algorithms: “When these systems fail, they fail most the people who are already marginalized.”
Synthetic content
A recent survey by GLG found that 87 percent of companies do not identify chatbots as such, instead allowing users to assume that they are communicating with another human being. There is barely any industry disclosure about the use of simulated training data in human training or AI training situations. And, of course, there are deepfakes, which are not disclosed at all and simply make the rounds until someone manages to debunk them.
The ethical issues with synthetic content, not to mention potential criminal applications such as blackmail, framing, and security penetration, are so great that some jurisdictions are already clamping down on its use. The state of California, for example, has banned the use of bots to impersonate human beings for commercial or political purposes.
But what can we do about these?
The technology may be here to stay, but that doesn’t mean we have to accept the ethical problems that come with it, Baxter said. She offered a few suggestions for companies that are serious about ensuring that their use of AI does not compromise ethics: firstly, think of bias in terms of statistical error, and mitigate it accordingly. Secondly, don’t be afraid to take down implementations of AI if the bias in the system cannot be fixed, as Amazon did. Thirdly, don’t just focus on the model: look at whether the outcome is ethically correct, because even a semantically correct model can produce profoundly inequitable outcomes.
And most importantly, she said, do not get into the mindset of a race to the bottom. Pointing to the often-cited fear that China’s AI capabilities will outstrip the US, she said: “So what if China’s facial recognition is dramatically more accurate than in the US? We can’t use how another company or country is acting to justify violating human rights.”